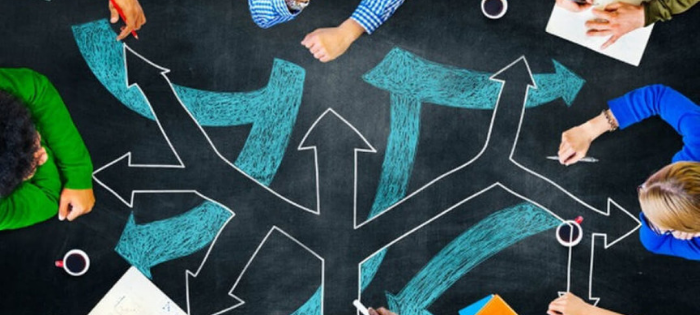
Environment / Quality / Safety
Comments: No Comments
Today’s business managers face greater complexities than ever when it comes to making business decisions. For every business decision, there are a number of factors that impact the associated risks. Fortunately, the use of statistics, predictive analytics, and data mining has become increasingly useful in taking the “gut feel” out of making important and often complex business decisions.
Data-Driven Decisions
Most people are familiar with common descriptive statistical techniques, like measures of central tendency (e.g., mean, median, mode) or variability (e.g., interquartile range, standard deviation). More advanced data mining and predictive analytical techniques are increasingly being used to explore and investigate past performance to gain insight for future business decision making.
Data mining draws on large amounts of data to identify patterns, which are often classified as opportunities or risks. Predictive analytics encompasses a variety of statistical techniques that are used to analyze historical data to predict the most probable future events. A few examples of these include the following:
- Discriminant Analysis – a machine learning model where a computer program “learns” a pre-existing data set that includes attributes and outcomes for each individual, and then predicts probable outcomes for individuals in the new data set based on attributes.
- Linear Regression – creates an equation so that one variable can be predicted based on the known values of other variables.
- Logistic Regression – a machine learning model where a computer program “learns” a pre-existing data set that includes attributes and a binary (“yes/no”) outcome for each individual, then predicts “yes/no” outcome for each individual in a new data set, along with a probability associated with the decision.
- Decision trees – machine learning model where a computer program “learns” a pre-existing data set that includes attributes and outcomes (not necessarily binary) for each individual, then predicts outcomes for each individual in a new data set, along with confidence in the decision; also identifies the attributes that are most helpful for making predictions (i.e., those that are best able to discriminate between outcomes).
- Neural networks – similar to decision tree, but more effective if finding the connections between attributes is a concern.
Together, this information can help decision makers to predict the outcome(s) of a decision before it is made—and make smarter decisions based on data instead of gut feelings. The following case studies demonstrate the value that statistics provide when it comes to making important business decisions.
Case Study: Wildfire Risk Index
For a large transportation organization, wildfires have historically presented a unique challenge. The company has worked diligently over the past several years to control its fire risk through research and a number of assessments. To help further minimize the wildfire risk, the company turned to past data and is working with Kestrel to develop a comprehensive Wildfire Risk Index to:
- Quantify the operational risks of wildfires (i.e., identify environmental conditions, determine areas of concern)
- Make informed business decisions to help minimize identified risks
Creating the Index requires a significant amount of data from both internal and external resources, including traffic, weather, geography, internal fire incidents, and others. This information is used in several components contained within two main models that create the Wildfire Risk Index. These model components are relatively simple when used on their own. The complexity arises when combining the various models and their components into a single Wildfire Risk Index that reasonably reflects relative risks, while considering all variables.
The ultimate output of the Wildfire Risk Index is a single number that quantifies the relative risk of wildfire by location and by month. This information will help the company to:
- Identify the areas of greatest risk.
- Focus resources on those areas.
- Make more informed decisions regarding operations—like when to plan hot work and when and where to perform vegetation control—to help prevent future incidents.
Case Study: Incident Data
For a large petroleum refining organization, safety and environmental incidents present a significant risk to operations. In order to reduce incident frequency, the company has implemented a robust safety management system, which includes frequent audits and inspections. Despite the company’s best efforts, however, incidents have continued to occur.
To further improve safety and environmental performance, Kestrel is working with the company to conduct detailed reviews of previous incidents using Kestrel’s proprietary Human Performance Reliability (HPR) approach. This approach identifies and classifies the human factors contributing to incidents, as well as the controls associated with those human factors (engineered, administrative, and/or PPE). Once the reviews are finished, the results are statistically analyzed to generate a prioritized list of human factors to be addressed. Kestrel’s Human Factors Integration Tool (HFIT™) software then generates a list of existing controls associated with the top human factors, as well as a list of missing controls that could be created and implemented.
The ultimate output of the incident review process is to help the company identify the human factors contributing to incidents, create or improve associated controls, manage operational risks, and protect the health and safety of workers and the surrounding environment.
Versatility
These examples demonstrate how predictive analytics can be used to support decision making. The versatility of predictive analytics, combined with the variety of statistical techniques available, can be applied to help companies analyze a wide variety of problems and gain insight for future business decision making.