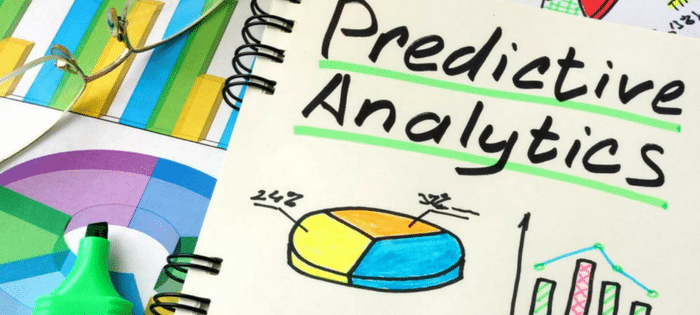
Safety
Comments: No Comments
Companies are generating ever increasing amounts of data associated with business operations, leading to renewed interest in predictive analytics, a field that analyzes large data sets to identify patterns, predict outcomes, and guide decision-making. Companies are also facing a complex and ever expanding array of operational risks to proactively identify and mitigate. While many companies have begun using predictive analytics to identify marketing/sales opportunities, similar strategies are less common in risk management, including safety.
Classification algorithms, one general class of predictive analytics, could be particularly beneficial to the refining and petrochemical industries by predicting the time frame and location of safety incidents based on safety related inspection and maintenance data, essentially leading indicators. There are two main challenges associated with this method: (1) ensuring that leading indicators being measured are actually predictive of incidents, and (2) measuring the leading indicators frequently enough to have predictive value.
Kestrel’s article in the Q3 2018 edition of Petroleum Technology Quarterly (PTQ) features a case study to illustrate this process. Using regularly updated inspection data, the author developed a model to predict where broken rails are likely to occur in the railroad industry. The model was created using a logistic regression modified by Firth’s penalized likelihood method, and predicts broken rail probabilities for each mile of track. Probabilities are updated as additional data are collected.
In addition to predicted broken rail probabilities, the model identifies the variables with the most predictive validity (those that significantly contribute to broken rails). Using the model results, the railroad was able to identify exactly where to focus maintenance, inspection, and capital improvement resources and what factors to address during these activities. Validation tests of the model revealed 70% of the actual broken rail incidents occurred on the 20% of segments at highest risk for broken rails.
The same methodology could be used in the refining and petrochemical industries to manage risks by predicting and preventing incidents, provided that organizations:
- Identify leading indicators with predictive validity
- Regularly measure leading indicators (inspection, maintenance, and equipment data)
- Create a predictive model based on measured indicators
- Update the model as data are gathered
- Use the outputs to prioritize maintenance, inspections, and capital improvement projects and review operational processes/practices.